How To Say Lack Of Data
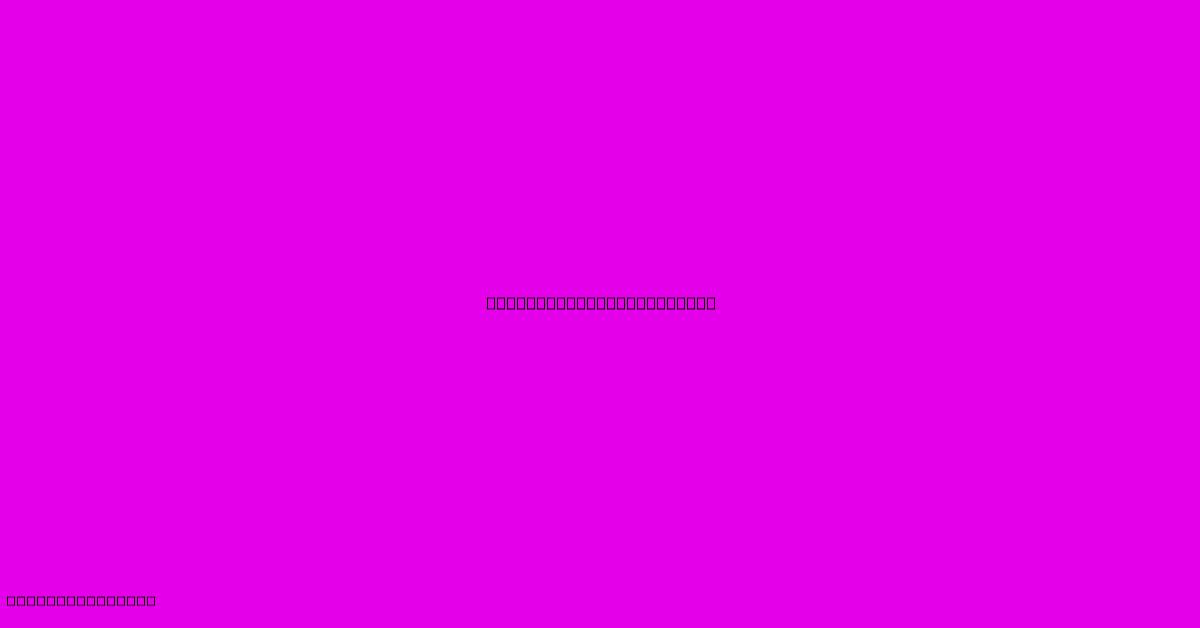
Discover more in-depth information on our site. Click the link below to dive deeper: Visit the Best Website meltwatermedia.ca. Make sure you don’t miss it!
Table of Contents
How to Say "Lack of Data": Navigating Data Scarcity in Research and Analysis
Hook: Does insufficient data derail your projects? A robust understanding of how to articulate and address data scarcity is crucial for impactful research and accurate analysis.
Editor's Note: This guide on effectively communicating "lack of data" has been published today.
Why It's Important & Summary: The absence of sufficient data is a common challenge across diverse fields, from scientific research to business analytics. Effectively communicating this scarcity is vital to maintain credibility, avoid misinterpretations, and guide decision-making. This article explores various ways to describe data limitations, methods to mitigate their impact, and strategies to strengthen future data collection. Relevant keywords include data scarcity, missing data, incomplete data, data limitations, data gaps, insufficient data, data deficiency.
Analysis: This guide draws upon established research methodologies, statistical practices, and communication best-practices to offer a comprehensive approach to handling data limitations. The information presented aims to empower researchers and analysts to transparently and effectively address data scarcity in their work.
Key Insights:
- Acknowledge the limitation directly and transparently.
- Quantify the extent of missing data when possible.
- Explore potential biases introduced by data scarcity.
- Employ appropriate statistical techniques for incomplete datasets.
- Propose strategies for future data collection.
How to Say "Lack of Data": A Comprehensive Guide
Subheading: Addressing Data Scarcity in Research and Analysis
Introduction: Data scarcity poses significant challenges in research and analysis. Effectively communicating the extent and implications of missing data is crucial for maintaining the integrity and validity of findings. This section explores various ways to articulate the presence of insufficient data and mitigate its impact on conclusions.
Key Aspects:
- Directly stating the data limitation: Using phrases like "limited data availability," "insufficient data points," or "incomplete dataset" provides a clear and unambiguous acknowledgement of the issue.
- Quantifying the data gap: Whenever possible, specifying the percentage or number of missing data points strengthens the description. For instance, "analysis is limited due to 25% missing data in the control group."
- Highlighting potential biases: Data scarcity can introduce bias, impacting the generalizability of findings. This should be explicitly acknowledged. For example: "The limited sample size may lead to a biased representation of the population."
- Employing appropriate statistical methods: Using techniques designed for handling incomplete datasets, such as imputation or multiple imputation, can partially mitigate the effect of missing data. These methods should be clearly explained.
Subheading: Discussing Missing Data: Methods and Implications
Introduction: This section examines specific strategies for addressing missing data, considering their implications for analytical validity and interpretation.
Facets:
- Types of Missing Data: Understanding the mechanism of missing data (Missing Completely at Random (MCAR), Missing at Random (MAR), Missing Not at Random (MNAR)) is essential for choosing appropriate handling methods. MCAR implies the missing data is random and unrelated to any other variables. MAR indicates that missingness is related to observed data but not to the missing data itself. MNAR, the most challenging type, suggests that missingness is related to unobserved data or the missing data itself. Understanding these distinctions is crucial for choosing an appropriate approach.
- Imputation Methods: Various techniques can fill in missing values. Single imputation replaces each missing value with a single estimate (e.g., mean, median, mode), while multiple imputation generates multiple plausible imputed datasets, allowing for an assessment of uncertainty related to the imputation process. The choice of imputation method should be justified based on the type of missing data and the research question.
- Sensitivity Analyses: These assess how sensitive the results are to the handling of missing data. Conducting sensitivity analyses using different imputation methods or assuming different missing data mechanisms helps to determine the robustness of the findings.
- Limitations of Imputation: Imputation methods can introduce bias, especially if the missing data mechanism is not properly understood. Therefore, transparent reporting of the imputation methods used and the limitations of the approach are essential.
Subheading: Proactive Data Management for Future Studies
Introduction: Addressing past data limitations requires planning for future data collection. This section explores strategies to improve data quality and reduce data scarcity in future research endeavors.
Further Analysis: Effective strategies include improving sampling techniques to enhance representativeness, establishing robust data collection protocols to minimize missing data, and utilizing data cleaning and validation processes to ensure data accuracy. Collaborations across multiple research groups or the development of comprehensive databases can improve data availability in particular fields.
Closing: By proactively addressing potential data limitations during the planning phase of research, researchers can significantly improve the quality and reliability of their findings. A thorough understanding of missing data mechanisms and appropriate handling techniques is paramount for generating robust and credible results.
Subheading: FAQ
Introduction: This section addresses frequently asked questions regarding data scarcity and its handling.
Questions:
- Q: What if I have a significant amount of missing data? A: With extensive missing data, the reliability of analysis is significantly reduced. Consider alternative research designs, data sources, or the feasibility of the project itself. Explore more sophisticated imputation methods that explicitly model the missing data mechanism, such as multiple imputation.
- Q: How do I justify my choice of imputation method? A: Justify the method based on the type of missing data (MCAR, MAR, MNAR), the characteristics of the data, and the research question. Transparency in reporting the chosen method and its limitations is crucial.
- Q: What are the ethical considerations of dealing with missing data? A: Ensure transparency and avoid misleading interpretations. Clearly state any limitations imposed by missing data and acknowledge potential biases.
- Q: Can I still publish research with limited data? A: Yes, but the limitations must be clearly articulated. Focus on the strengths of the research, the insights gained despite data limitations, and suggest avenues for future research to address the data gaps.
- Q: How do I avoid data scarcity in future research? A: Plan your data collection meticulously, implement robust data quality checks and validation steps, and consider alternative data sources.
- Q: What software tools are available for handling missing data? A: Several statistical software packages (e.g., R, SPSS, SAS) offer tools for handling missing data, including imputation and analysis of incomplete datasets.
Summary: The effectiveness of any research hinges on the quality and quantity of available data. Understanding how to appropriately address data scarcity is essential for maintaining research integrity, accurately interpreting findings, and planning future studies.
Subheading: Tips for Handling Data Scarcity
Introduction: These tips offer practical guidance on navigating challenges related to insufficient data.
Tips:
- Plan thoroughly: Develop a robust data collection plan, specifying sample size requirements and data quality controls.
- Explore alternative data sources: Consider using publicly available datasets, administrative data, or combining data from multiple sources.
- Employ robust data cleaning techniques: Thoroughly clean and validate your data to identify and address inconsistencies or errors.
- Use appropriate statistical methods: Select statistical methods designed for handling incomplete datasets, like multiple imputation.
- Conduct sensitivity analysis: Evaluate how different data handling techniques affect your conclusions.
- Be transparent: Clearly articulate data limitations and their potential impact on findings.
- Collaborate: Partner with researchers or organizations that have access to relevant data.
- Prioritize data quality over quantity: While sufficient data is crucial, prioritizing data quality ensures reliable findings even with a smaller dataset.
Summary: Implementing these tips enhances the robustness of analyses performed with limited data.
Closing Message: Effectively communicating the presence and implications of data scarcity is a critical skill for researchers and analysts. By embracing transparency, utilizing appropriate statistical methods, and planning for robust data collection in future studies, researchers can mitigate the impact of data limitations and produce reliable and impactful findings. Proactive data management and a thorough understanding of handling incomplete datasets are essential for generating credible and impactful research.
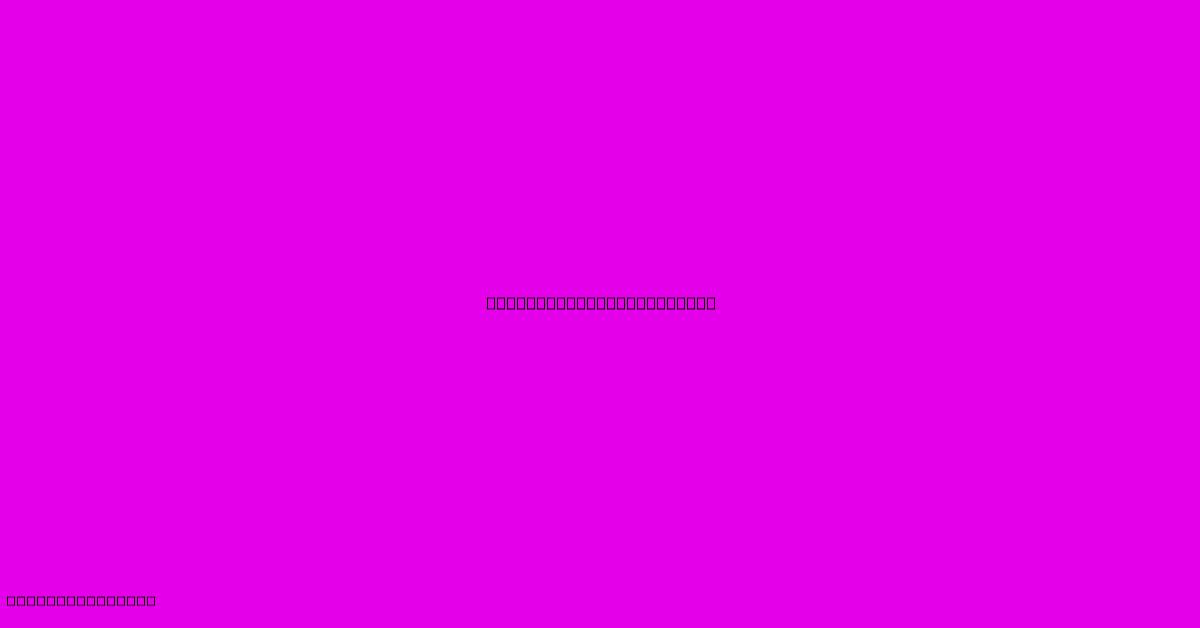
Thank you for taking the time to explore our website How To Say Lack Of Data. We hope you find the information useful. Feel free to contact us for any questions, and don’t forget to bookmark us for future visits!
We truly appreciate your visit to explore more about How To Say Lack Of Data. Let us know if you need further assistance. Be sure to bookmark this site and visit us again soon!
Featured Posts
-
How To Say Draw In Portuguese
Feb 04, 2025
-
How To Say Baby Girl In Korean
Feb 04, 2025
-
How To Say I Am Okay
Feb 04, 2025
-
How To Say Chilocco
Feb 04, 2025
-
How To Say I Read In Past Tense
Feb 04, 2025